Artificial Intelligence (AI) is rapidly transforming various industries, including the pharmaceutical sector. Regulatory authorities are increasingly exploring AI-driven tools to enhance decision-making processes related to drug and biological product approvals. However, the integration of AI in regulatory science requires careful consideration to ensure transparency, reliability, and compliance with established guidelines.
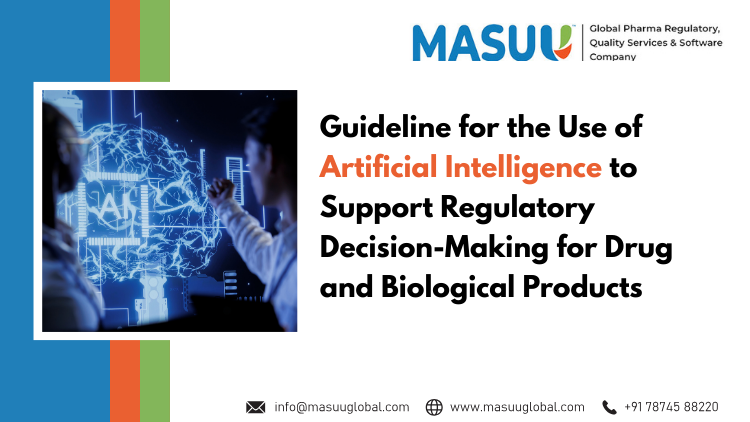
on the use of artificial intelligence (AI) to produce information or data intended to support regulatory decision-making regarding safety, effectiveness, or quality for drugs.
AI refers to a machine-based system that can, for a given set of human- defined objectives, make predictions, recommendations, or decisions influencing real or virtual environments.
A subset of AI that is commonly used in the drug product life cycle is machine learning (ML). ML refers to a set of techniques that can be used to train AI algorithms to improve performance at a task based on data. Although ML is currently the most utilized AI modeling technique in the drug product life cycle, this guidance focuses on AI models more broadly.
Continuous advancements in AI hold the potential to accelerate the development of safe and effective drugs and enhance patient care.
The risk-based credibility assessment framework outlined here follows a 7-step process designed to evaluate and establish the credibility of an AI model’s output for a specific Context of Use (COU) based on its associated risk.
The Focus of the GuidanceThis draft guidance focuses on the application of AI models that produce data or insights supporting regulatory decisions on drug safety, effectiveness, and quality. It does not cover AI applications in drug discovery or operational processes that do not directly impact patient safety, product quality, or study reliability. The recommendations apply throughout the entire drug lifecycle, including preclinical research, clinical trials, postmarket surveillance, and manufacturing.
A Seven-Step Risk-Based Framework for AI CredibilityTo establish the credibility of AI models in regulatory contexts, the FDA proposes a seven-step risk-based framework:
- Identify the Regulatory Question
- Specify the Context of Use (COU)
- Evaluate AI Model Risk
- Model Influence: The degree to which the AI model’s output contributes to regulatory decisions.
- Decision Consequence: The potential impact of incorrect decisions based on the model’s results.
- Develop a Credibility Assessment Plan
- Implement the Plan
- Record and Report Results
- Assess Model Suitability
- Reduce the model’s influence by integrating additional data.
- Strengthen validation processes.
- Implement risk mitigation strategies.
- Refine the model or adjust methodologies.
- Continuously reassess the model until it meets the required standards.
Because AI models can evolve, the guidance stresses the need for continuous monitoring, regular evaluations, and timely updates. A risk-based approach should guide ongoing lifecycle management to maintain consistent and accurate performance.
Encouragement for Early FDA CollaborationThe FDA strongly recommends that sponsors engage in early discussions when developing AI models for regulatory use. Early collaboration helps define clear validation expectations and proactively address potential challenges. The agency provides multiple engagement opportunities, including formal meetings and consultative programs.
Potential Industry ImpactThis guidance is set to have a profound effect on pharmaceutical and biotech companies by promoting responsible innovation and establishing clear pathways for AI integration. Early adopters of these standards can gain a competitive edge by optimizing regulatory processes while maintaining compliance.
Challenges and Considerations for ImplementationAdopting this framework may pose challenges, such as validating AI models, safeguarding data privacy, and seamlessly integrating AI into existing regulatory and operational workflows. To meet regulatory expectations, organizations must invest in rigorous validation measures and establish continuous monitoring processes.
Global Regulatory ContextThe FDA’s approach to AI regulation aligns with growing global efforts to manage AI use in healthcare. Comparing this guidance to international standards, such as those from the European Medicines Agency (EMA) and the UK’s Medicines and Healthcare products Regulatory Agency (MHRA), can offer a broader understanding of global regulatory trends in AI applications for drug development.
Future ProspectsAs AI technology advances, its role in regulatory decision-making will continue to expand. Agencies must proactively adapt to technological developments by fostering research collaborations, updating regulatory guidelines, and investing in AI literacy among regulatory professionals.
The successful integration of AI in regulatory science depends on a balanced approach that prioritizes transparency, accuracy, and ethical responsibility. By addressing these key considerations, AI can significantly improve the efficiency and effectiveness of drug and biological product regulation, ultimately benefiting public health and innovation in the pharmaceutical industry.
ConclusionThe FDA’s draft guidance offers a comprehensive framework for integrating AI into regulatory decision-making in drug and biologic development. By emphasizing a risk-based approach, transparency, and lifecycle management, the guidance ensures that AI is applied responsibly, balancing innovation with the highest standards of safety and efficacy throughout the drug development process.
References: https://www.fda.gov/media/184830/download